Key Takeaways
- Sensors, cameras and open-source IP are making it easier to harness data at scale.
- Three distinct data strategies are focused on expanding opportunities for data extraction, data refinement and data distribution.
- Data needs to go through a series of steps to derive actionable insights: execute, modify and transform.
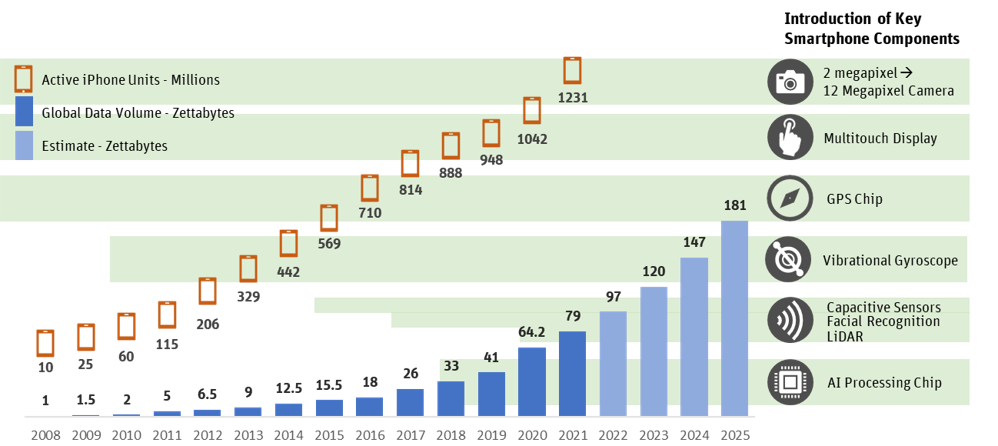
Source: IDC, Apple, SVB Analysis
By 2025, the International Data Corporation forecasts the global volume of data will grow to 181 zettabytes. (One zettabyte equals one trillion gigabytes). To put this growth in perspective, the world’s collective internet usage reached one zettabyte in 2016, according to Cisco. In 2011, Marc Andreessen coined the phrase “software is eating the world,” while at the same time, Peter Thiel famously stated, “We wanted flying cars, instead we got 140 characters,” and they were both right. Historically software has been primarily successful at consuming clean structured data, not the noisy, unstructured data inhabiting most of our world.
Massive physical industries (like construction, manufacturing and agriculture) have been slower to capture and harness data at scale. This is changing rapidly thanks to several enabling influences.
Sensors
Sophisticated sensors and related components have become more affordable. For example, the iPhone 12’s LiDAR sensors use semiconductor fabrication techniques to greatly reduce cost. Compared to a decade ago, the first three-dimensional LiDAR sensor, built by Velodyne cost $75,000. The technology has gone from the realms of large enterprise to the everyday consumer.
Cameras
The camera on the back of a smartphone has sparked the invention of apps like Instagram and TikTok. The same underlying technology that allows scientists to take satellite images of Earth from space also enables surgeons to perform medical imaging to treat their patients. Hardware-based companies and the data they capture are becoming both a commercialized product and training set for machine learning.
Access to intellectual property
IP is now open source. NASA releases its patents into the public domain via its technology transfer program. Stanford’s RoboTurk, Google’s ClearGrasp and Amazon’s TEACh have all released datasets on object manipulation and commands to encourage commercialization.
Having access to massive training data sets and the latest open-source AI techniques, complemented by increased processing power in chips and quickly decreasing materials costs, allows startups to acquire new, unique and highly differentiated data sets of our physical world. These complex data sets require strategies for data extraction, data refinement and data distribution.
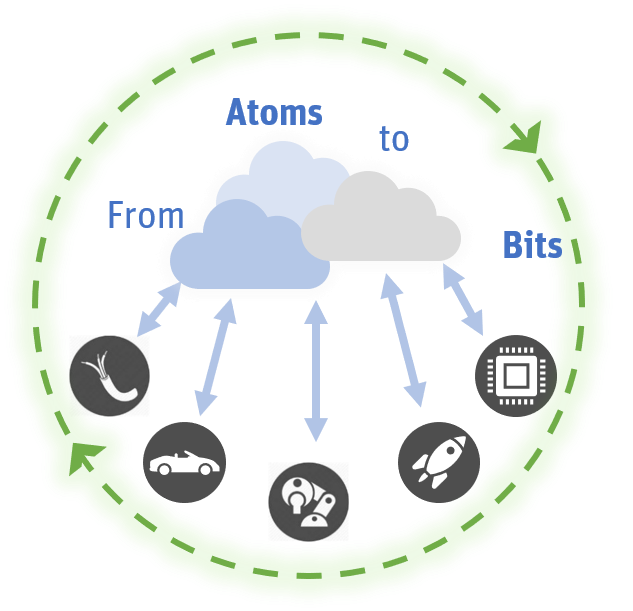
Three data strategies
Defining companies working on deep tech technologies based on engineering innovation and scientific research, can be complex. So, to simplify matters, we’ve separated company profiles into three distinct data strategies: The Moonshot, The Pick & Shovel and The Wedge. Often firms fall squarely into one bucket while others will blur the boundaries. Each type of firm has a different relationship with data.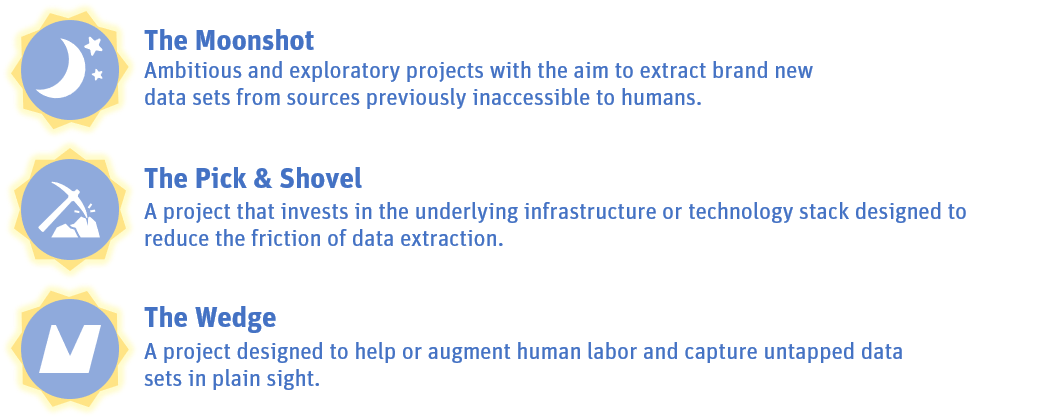
The moonshots
Moonshots typically take a long time to come to market. They are exploratory, trying to accomplish something that has likely never been attempted. If they do succeed, proprietary data can be captured that would have previously been unattainable. The private space industry has gone from science fiction to reality over the past two decades. Planet Labs (NYSE: PL) and Spire Global (NYSE: SPIR) have built successful business models providing satellite data to a variety of industries: agriculture, maritime, energy, environment and governments. Both companies spent years – with little to no revenue – building businesses that broke technological boundaries. Planet created a satellite constellation that could deliver earth imaging at a high refresh rate – the same imager viewed in Google Maps. The EU and US government use Spire Global’s climate data for weather monitoring. Moonshots have high capital expense barriers to entry but with the opportunity to further develop data marketplace offerings or partner with third parties.
The pick and shovel
Pick and Shovel firms are the infrastructure businesses necessary for deep tech to progress. They include specialized chip manufacturers, powering data processing for AI and machine learning applications. For example, Mythic is a startup focused on building small, yet powerful GPUs that enable devices for surveillance, manufacturing and automotive to have the computing power once reserved for cloud computing. Devices using this type of edge AI technology are at “the edge” – outside of the centralized data centers in the cloud. Companies supplying chips, sensors, algorithms, software, tools, subsystems and services are linking up via partnerships such as The Edge AI and Vision Alliance. Well-positioned Pick and Shovel firms will benefit from riding the ever-growing wave of demand for more data about our physical world.
The wedge
Wedge companies quickly bring technology solutions to the front lines using off-the-shelf technologies. For example, Simbe Robotics has built a retail inventory tracking robot leveraging commercially available components such as Intel’s Realsense 3D cameras and Nvidia’s GPU platform. In 2017, they began piloting their inventory tracking robot, Tally, with Schnucks, a midwestern grocery chain. Using Tally’s data, retailers can adjust their buying strategy to improve fill rates for orders, decrease substitutions and avoid stockouts. Wedge companies build both the hardware and operating software, monetizing first on execution of a basic task. The data they acquire adds monetization opportunities either directly or through data agreements.
No matter the data strategy, all three models are collectively focused on expanding opportunities for data extraction, data refinement and data distribution. Or better said, converting atoms to bits.
Progressing from atoms to bits
Data needs to go through three steps to derive actionable insights. Not all companies will require each step.
Execute
In 2021, more than 47 million people in the United States quit their jobs. Hard hit were durable goods manufacturers, wholesalers and retailers—industries that already incorporated automation technologies. Now, as companies feel the worker pinch, they are increasing their investment in automation and expanding its scope of operations. The benefits can be huge. Simbe Robotics Tally robot’s first use case was in February 2020, monitoring on-shelf availability (OSA). The more time the robot operates, the more its performance improves, a characteristic of technologies that use reinforcement machine learning algorithms. Tally can scan between 15,000-30,000 items per hour at 97% accuracy versus a human who would take a full week to do the same task at 65% accuracy.

Modify
In March 2020, at the start of the COVID-19 pandemic, Simbe Robotics observed, via data from its deployed Tally robots, out of stocks jumped to 11%. (A normal range is 3–6%). How much of this could be attributed to factors out of a store manager’s control? Of the out of stocks Tally detected, 20–30% were not related to lack of warehouse inventory, rather in-store inefficiencies such as delayed restocking.

Transform
As a company expands its network of customers, they can commercialize this data set further. Hardware companies are starting to get savvy about establishing data sharing agreements with their customers. In many cases, this can be a symbiotic relationship where data collected from a machine can be useful to a third party. The proceeds of selling this data can be shared by the robotics company and their customer.
The impact of smartphone advancement
The adoption of smartphones, advancement of the underlying technology, plus the addition of more and more features has exponentially increased the quantity and types of data collected. The smartphone has become a platform for previously unconceived or impossible-to-create applications. Many businesses like Uber, Lyft, Snapchat, Venmo and Niantic are solely reliant on the sophistication and prevalence of smartphones and the rich data they collect. For investors, this was a big draw as software applications could be developed fast, didn’t require significant capital expenditure and had a steep adoption curve (if successful).With changes to data privacy, personified by Apple’s iOS 15 update, the accessibility of data and concerns around privacy have meant companies that solely rely on third-party data face significant risks.
Investors are beginning to recognize the advantage of companies having control over data collection, namely through solutions with an integrated hardware component.
As such, I expect the prevalence of integrated hardware solutions in market to increase as companies look to secure the process of converting atoms to bits. This includes tapping new data sources and expanding the types of data collected. Who knows what insights will come from converting atoms to bits, or who will benefit most from their critical insights, but I am confident that a world with more data to drive decisions, improve transparency or build general knowledge will be a better one.